As the world electrifies, new demands are placed on the battery. Clean energy must have the same performance transparency as traditional power sources burning fossil fuel, but we realize that battery diagnostics is lagging behind. Without mature ways to assess battery state-of-health (SoH), battery users ask: “Will my battery die quietly or quit with a bang?” Lesson learned reminds us of the railway tracks laid across the continent in mid-1800 that were too frail and needed replacement with double the ties to carry heavy trains traveling at high speed. |
![]() |
Aviation also learned a lesson with early jet travel. In the 1950s, the elegant Comet developed cracks at rivet points around window frames after 9,000 flying hours that caused the fuselage to disintegrate and the plane to crash. Reinforcement with round windows fixes the problem with modern planes.
![]() |
![]() |
In the 1960s, industry made milestone by introducing Reliability-centered Maintenance (RCM) with strategic maintenance schedules on machinery. RCM was first applied on the Boeing 747 to improve air safety. These practices then spilled into other industries, such as nuclear plants, defense, and railways.
Batteries for critical uses require innovative testing to fully utilize each battery and replace them before failure. One of these test methods is electrochemical impedance spectroscopy (EIS), also known as Spectro™. A frequency scan produces a Nyquist plot representing the electrochemical evidence of a battery. The signature is compared with a matrix of batteries of the same model with varying performances, a concept used in face recognition using AI. The frequency scan ranging from 2,000Hz to 4Hz is minimally invasive. Test time is 30 seconds, longer with a large battery with an extended scan down to 0.1Hz. |
![]() |
![]() |
The scanned data goes through filtering and magnitude extraction. Modelling and data fusion correlate the values to derive capacity, CCA, SoC and other readings. Matrices are available for lead acid and Li-ion batteries. |
Capacity estimation of portable Li-ion batteries is mostly done by a System Management Bus (SMBus). With Usable Battery Energy known, the battery user must now establish the Minimum Operational Reserve Energy (MORE). Deep Battery Diagnostics (DBD) makes this possible by setting the Target Selector to a capacity threshold that delivers sufficient Leftover Charge for a given daily routine. DBD also evaluates predictive fade based on manufacturer’s specifications. Compiling the data as illustrated in Figure 5 leads to RUL, the ultimate disclosure to battery users.
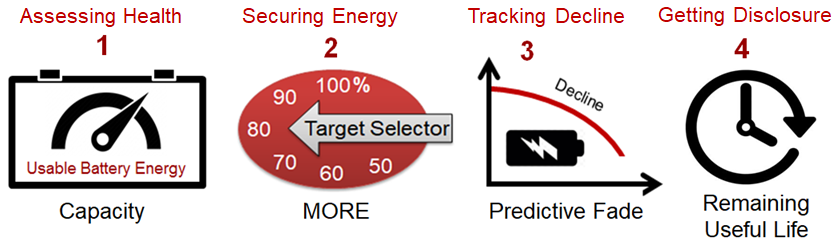
The DBD algorithm can be built into service devices, such as chargers, battery analyzers and monitoring systems serving batteries for portable, mobile, auto and stationary applications.
Modern battery chargers are no longer built to work in standalone mode. Figure 6 illustrates connecting the charger or analyzer to a host in Level 2 and Cloud Analytics in Level 3 to form a powerful battery supervisory servicing smart batteries. Capacity assessment with “dumb” batteries is also possible with the parser technology.
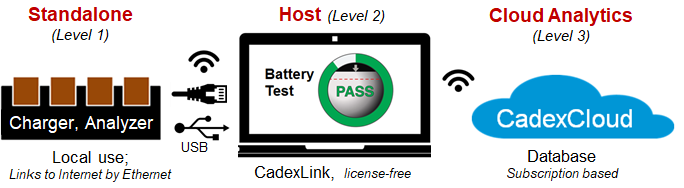
Modern battery-care systems must be cost-effective and simple to operate. Figure 6 illustrates functions of a fully extended system starting with the lone charger. Scalability makes a battery fleet transparent with ability to set the ‘sweet spot’ between high reliability and long battery life.
![]() |
User: Level 1 |
![]() |
![]() |
Supervisor: Level 2 |
![]() |
![]() |
Cloud Analytics: Level 3 |
![]() |
The Fishbowl makes battery state-of-health transparent with Leftover Charge marked in percentage. The outer ring reveals state-of-charge that moves clock-wise with charge and counter-clockwise with discharge. The hanging black ceiling tracks capacity that slowly drops towards the set Target Selector redline as the battery ages. Touching the Status Dome displays battery data that includes battery manufacturing date, ratings, as well as cell mismatch with some smart batteries. A status message discloses the readiness of a battery. |
![]() |
![]() |
Leftover Charge is set by the Target Selector. An analogy is an aircraft carrying enough fuel for landing. If Leftover Charge is low, the target capacity should be set higher to increase reserve energy for a given application. If consistently high, the setting can be lowered to keep batteries in service longer. |
Recommended target capacity settings of fleet batteries are:
85-90%: Critical mission; fewer batteries will pass
80%: Default; golden average that works for most uses
60-70%: Relaxed duty; batteries can be kept in service longer
Modern chargers with Target Selector may be set to different settings. For example, 90% will only pass batteries for critical uses. Once the capacity reduces, the battery is placed into the 80% charger for general use. With further fade, this battery is then kept in the 70% charger for less critical uses. Such a system lowers cost of ownership without sacrificing system reliability und lowering environmental concerns.
Conclusion
A DOE official said: “Every year roughly one million usable lithium-ion batteries are sent for recycling.” The US Army also discards tons of batteries, in part due to inactivity. Roughly 75% of vehicles on standby are driven less than 3,000 miles per year, causing battery sulfation, a film buildup that occurs when a lead acid battery is deprived of a full charge. Sulation can often be reversed if serviced in time.
Records disclose that batteries in armored vehicles were being replaced every 13 months on average, breaking the expected life expectancy of 6 to 8 years. In fiscal 2016 alone, the Department of Defense replaced more than 373,000 batteries in a pool of 400,000 at a cost of over $80 million. (See https://asc.army.mil/web/news-alt-ond17-harnessing-the-sun/ Technology is available that assess the condition of a battery and applies corrective service to put packs back in service. Source: U.S. Army photo by Chip Herrell, |
![]() |
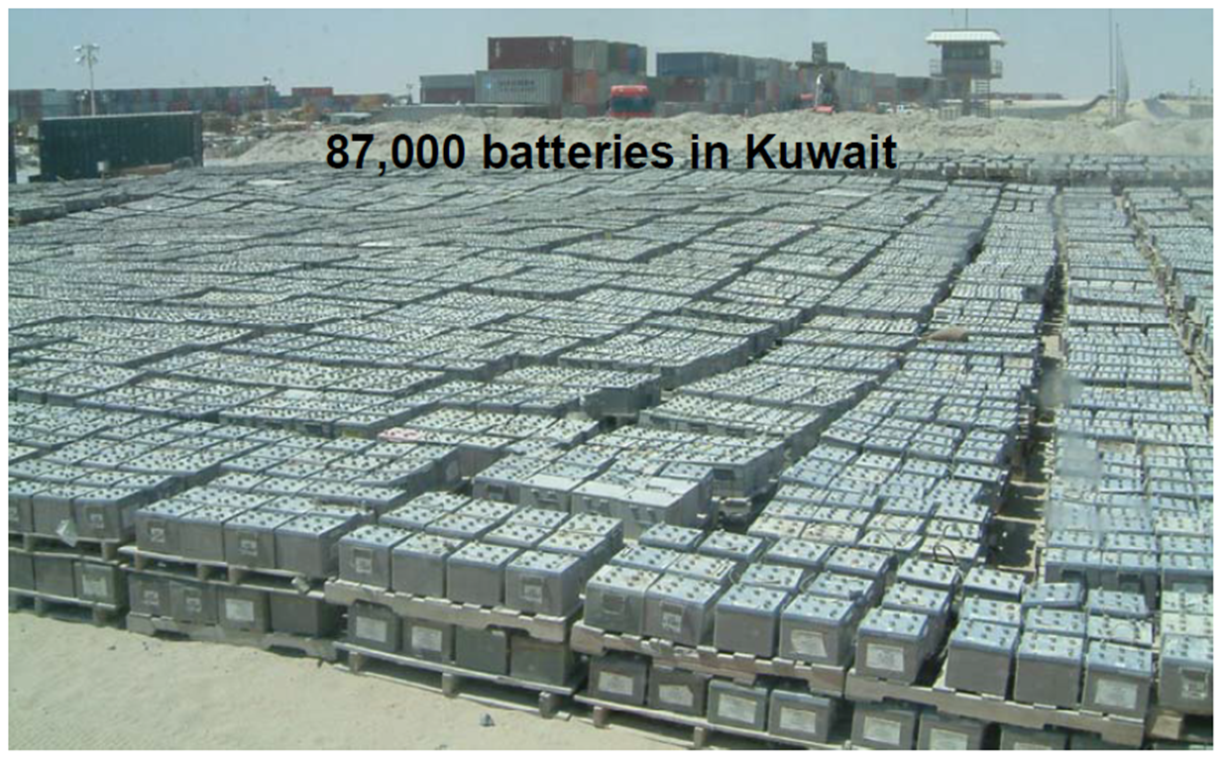
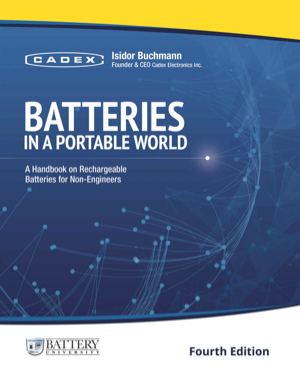